Getting started with Business Intelligence: practical tips
« Wisdom is about extracting gold from raw data; with sharp Business Intelligence, every piece of information becomes a nugget. »
This adage perfectly sums up the potential of BI, provided you follow a few practical tips. Existing information goldmines allow companies to turn them into nuggets of gold shaped in their own image.
Definition
Business Intelligence (BI) is a set of processes, technologies and tools used to collect, analyse, interpret and present data in order to provide actionable information to an organisation’s decision-makers and stakeholders. The main objective of BI is to help companies make strategic decisions based on reliable and relevant data.
BI is widely used in many areas of business, such as financial management, human resources management, marketing, sales, logistics and supply chain, among others. In short, Business Intelligence aims to transform data into actionable knowledge to improve an organisation’s overall performance.
Before looking at the practical tips, let’s look at the elements that define BI. To put BI into practice within your business, there are 5 main steps you need to follow to achieve relevant and effective BI.
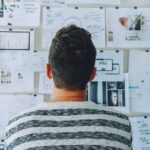
Data collection |
Data is collected from a variety of sources inside and outside the company, such as transactional databases, business applications, social media, customer surveys, etc. |
Data cleansing and transformation |
The data collected is cleaned, normalised and transformed into a format that is compatible for analysis. This often involves eliminating duplicates, correcting errors and standardising data formats. |
Data analysis |
Data is analysed using various techniques such as statistical analysis, data mining, predictive models and machine learning algorithms to identify trends, patterns and insights. |
Data visualisation |
The results of analysis are generally presented in the form of dashboards, reports, graphs and other interactive visualisations to facilitate understanding and decision-making. |
Informations dissemination |
The information obtained is shared with decision-makers and stakeholders throughout the organisation, enabling them to make informed decisions based on reliable data. |
Practical tips
Now that we have a broad understanding of the definition of BI, it’s important to remember that getting started with Business Intelligence (BI) can be a challenge, but with a strategic approach and some practical advice, you can put in place an effective infrastructure for your business.
Here are some practical tips for getting started with relevant and effective Business Intelligence.
Clarify your objectives
Before you start implementing BI, clearly identify the business objectives you want to achieve. Whether you want to improve decision-making, optimise business processes or better understand your customers, clear objectives will help you focus your efforts.
Start with the basics
Don’t try to do everything at once. Start with pilot projects or specific initiatives to familiarise yourself with BI concepts and tools. This will also enable you to measure results quickly and adjust accordingly.
Identify your data sources
Identify your organisation’s internal and external data sources. This can include transactional databases, spreadsheets, CRM systems, online marketing tools, etc. Ensure that the data you collect is reliable, complete and relevant to your objectives.
Clean and prepare your data
Data quality is essential for effective BI. Put processes in place to clean, standardise and prepare your data before analysing it. This often involves eliminating duplicates, correcting errors and standardising data formats.
Choose the right tools
There are many BI solutions on the market, so look for those that best suit your needs. Considers factors such as ease of use, the ability to manage large sets of data, integration with your existing systems and cost.
Train your team
Make sure your team is formed to use BI tools and interpretation of data. BI is a powerful tool, but its effectiveness depends on the ability of your team to use it properly.
Communicate and collaborate
Involve stakeholders from the start of the BI implementation process. Their support and comments will be essential to ensure the long-term success of your initiative BI.
Start small and grow
Don’t try to implement all BI functionalities at once. Start with pilot projects or specific initiatives, and then gradually extend your use of BI according to the results obtained.
Involve stakeholders
Involve stakeholders right from the start of the BI implementation process. Their support and feedback will be essential in ensuring the long-term success of your BI initiative.
Measure and adjust
Track the performance of your BI and measure its impact on your business. Use this information to identify areas for improvement and make adjustments to your BI strategy over time.
By following these initial practical tips, you can get off to a good start with Business Intelligence and start leveraging your data to make informed decisions and drive business growth.
CONCLUSION
A Business Intelligence (BI) project is considered successful when it succeeds in adding value to the business by meeting its business objectives effectively and efficiently. Here are some key indicators that can define a successful BI project:
Alignment with business objectives: the BI project must be aligned with the company’s strategic objectives. It must contribute to improving decision-making, optimising business processes, increasing profitability or strengthening the company’s competitiveness.
Effective use of data: a successful BI project makes effective use of data to provide usable information. This means collecting, cleansing, analysing and presenting data in the right way to meet business needs.
User adoption: end-users must adopt BI tools and use them on a regular basis to make decisions. A successful BI project is one that meets users’ needs and is easy to use and understand.
Improved performance: a successful BI project translates into improved business performance. This can take the form of increased sales, reduced costs, improved productivity or any other performance measure relevant to the business.
Positive return on investment (ROI): a successful BI project generates a positive return on investment for the business. This means that the benefits gained from using BI outweigh the costs of implementing and maintaining the project.
Scalability and flexibility: a successful BI project is capable of adapting to the changing needs of the business and evolving with it. It must be flexible enough to support new needs, new types of data or new usage scenarios.
Management support and commitment: a successful BI project benefits from the support and commitment of the company’s management. Management must recognise the value of BI and provide the necessary resources to support the project throughout its lifecycle.
In summary, a successful BI project is one that contributes to achieving the company’s business objectives by effectively using data to make informed decisions. It is characterised by its alignment with business objectives, its adoption by users, its positive impact on business performance and its positive return on investment.