DATA! DATA ! DATA everywhere!
These days, data is everywhere, featuring prominently in all new projects and corporate strategies. It’s the key to performance in these uncertain times. At Business Lab consulting, we’re the first to be convinced that it’s a powerful tool that accelerates performance…when it’s well used, well understood and well mastered!
In this new series of articles, we’re going to talk about the big bad wolf; the devil that hides in the detail (or sometimes reveals itself in broad daylight) and discuss with you the 7 main types of pitfalls posed by data and its use. As far as possible, we’ll try to illustrate them with an example from our own experience, because as experts we’ve had the good fortune to come up against each of them in our missions…
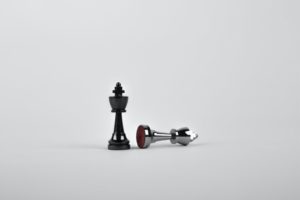
Note: these are the pitfalls discussed in Ben Jones’ book, “7 data pitfalls”, which we highly recommend!
Enough suspense, let’s now unveil the 7 families of DATA deadly sins that we’ll be exploring in greater detail over the next 7 weeks:
1. Epistemological errors: how do we think about data?
We often use data with the wrong frame of mind, or with erroneous preconceptions. So, if we go into an analysis project thinking that the data is a perfect representation of reality; if we draw definitive conclusions based on predictions without questioning them; or if we look in the available information for anything that might confirm an opinion already made; then we can create critical errors in the very foundations of these projects.
2. Technical errors: how are the data processed?
Technical and technological issues are often a major source of error in the world of data. Once you’ve identified the information you need, there’s a whole series of obstacles to overcome. Are my sensors working? Do my processes not generate duplicates? Is my data clean or up to date? Complex issues in our projects! After all, isn’t it said that a data analyst spends most of his time and energy preparing and cleaning his data?
3. Mathematical errors: how are the data calculated?
So now you know what your math lessons from school, college and high school are all about! There’s something for every level and taste! If you’ve never combined data at different levels of detail, or made mistakes when calculating ratios, or forgotten that you shouldn’t mix carrots and bananas, we’d love to hear from you!
4. Statistical errors: how are data related?
As the saying goes, “There are lies, damned lies and statistics”. This is the most complex trap to get to grips with, because it takes a lot of skill to fully understand what’s at stake. However, in a world where machine learning, datamining and AI are king, it’s a family of errors that’s only becoming more common!
Do the measures of central tendency or variation we use lead us astray? Are the samples we work with representative of the population we want to study? Are our comparison tools valid and statistically significant?
5. Analytical aberrations: how are the data analyzed?
So now you know what your math lessons from school, college and high school are all about! There’s something for every level and taste! If you’ve never combined data at different levels of detail, or made mistakes when calculating ratios, or forgotten that you shouldn’t mix carrots and bananas, we’d love to hear from you!
Golden rule: we’re all analysts (whether we have that title or not).
As soon as we use data to make decisions, then we are analysts, and therefore prone to making decisions based on aberrant analyses. For example, are you familiar with vanity metrics? Or have you ever made extrapolations that don’t make sense in the light of the data used?
6. Graphic blunders: how are data visualized?
Unlike statistical errors or analytical aberrations, graphical blunders are well known and easily identifiable. Why? Because they can be seen (often from a distance). Have we chosen the right type of chart for our analysis? Is the effect I want to show clearly visible?
7. Aesthetic hazards: can beauty be the enemy of goodness?
What’s the difference with graphic blunders?
Here we’re talking about the overall design of the final product and the interactions we’ve defined within it to ensure that the audience we’re trying to convince has the most ergonomic and aesthetically pleasing experience possible! Does the choice of colors we’ve made confuse or simplify the analysis? Have we used our creativity to make our dashboards pleasing to the eye, and have we used aesthetics to bring impact to the analysis we’re making? Is the final product easy to use and ergonomic, or are the interactions complex and time-consuming?
Are you ready to follow us through the twists and turns of everything that can go wrong with your data analysis projects, so that you don’t fall into these traps?
See you next week!
1 Comment