“There are lies, damned lies and statistics” B.Disraeli
Why such distaste for a field that, according to Webster’s Merriam-dictionary, is simply “a branch of mathematics dealing with the collection, analysis, interpretation and presentation of masses of numerical data. ”1 Why is the field of statistics in such a negative light by so many people?
There are four main reasons
- It’s a complex field. Even the basic concepts are not easily accessible and are very difficult to explain.
- Even the best-intentioned experts can misapply the tools at their disposal.
- The third reason behind all this hatred is that those with an agenda can easily create statistics to lie about when communicating with us.
- The final reason is that statistics can often seem cold and distant, making them very difficult for the public to grasp.
Descriptive setbacks
Descriptive statistics are intended to summarize the main characteristics of a data set. However, incorrect or inappropriate use can lead to misleading conclusions. A typical example is the use of the mean to summarize a distribution, without taking into account variability or skewness. Another common error is to present percentages without explaining the total number of people, which can be misleading as to the true extent of a phenomenon. It is therefore crucial to understand the assumptions and limitations of each descriptive measure in order to use it correctly.
Let’s take the example of analyzing salaries within a company. If we simply look at average salaries, we might conclude that the company is paying its employees well. However, if management salaries are very high compared to the rest of the employees, the average would be biased upwards. It would be more relevant to use the median, which gives the salary in the middle, or to look at the complete salary distribution for a more accurate view.
Inferential fires
Statistical inference aims to draw conclusions about a population from a sample of that population. However, this process is subject to error. Sampling errors and Type I and II errors are common. In addition, errors can be exacerbated by confusion between correlation and causation. A solid understanding of the principles of statistical inference is essential to avoid these pitfalls.
Let’s imagine a public health study seeking to establish a link between a particular dietary habit (such as eating organic) and better overall health. If the study finds a positive correlation, it doesn’t necessarily mean that eating organic causes better health. There could be confounding factors, such as income level or lifestyle, that influence both eating habits and health status. Here, we can fall into the trap of confusing correlation with causation.
Sliding sampling
Sampling is a crucial stage in any data collection process. Yet many errors can occur at this stage. The sample may not be representative of the target population, due to selection bias or non-response. What’s more, the sample size may be insufficient to detect an effect. Careful sample planning is therefore essential to obtain reliable results.
Consider a customer satisfaction survey conducted by an e-commerce company. If the company only solicits opinions from customers who have made a recent purchase, it runs the risk of obtaining a distorted picture of overall customer satisfaction. Indeed, dissatisfied customers may have stopped making purchases and therefore not be included in the sample. This is an example of selection bias.
Insensitivity to sample size
A common mistake in data analysis is to ignore the impact of sample size on results. A large sample size can make a very small effect significant, while too small a sample size may not have sufficient power to detect an existing effect. Furthermore, statistical significance does not necessarily mean practical significance. So it’s important to consider sample size when interpreting results.
Suppose you’re conducting a study to assess the effect of a drug on lowering blood pressure. If you have a very large sample of patients, you may see a statistically significant drop in blood pressure. However, this drop may be very small, say 0.1 mm Hg, a clinically insignificant value despite its statistical significance. This is an example where sample size can make a small effect significant. On the other hand, if the sample is too small, a real effect may be missed. It is therefore important to consider clinical or practical significance in addition to statistical significance.
Digging deeper into this issue, Ben Jones (see author who inspired this article) managed to find figures on kidney cancer rates as well as demographics for every US county, and he created an interactive dashboard (figure below) to visually illustrate the fact that Kahneman, Wainer and Zwerlink are doing quite clearly in words.
Notice a few elements in the dashboard. On the choropleth map (filled in), the darkest orange counties (high rates relative to the overall U.S. rate) and the darkest blue counties (low rates relative to the overall U.S. rate) are often side by side.
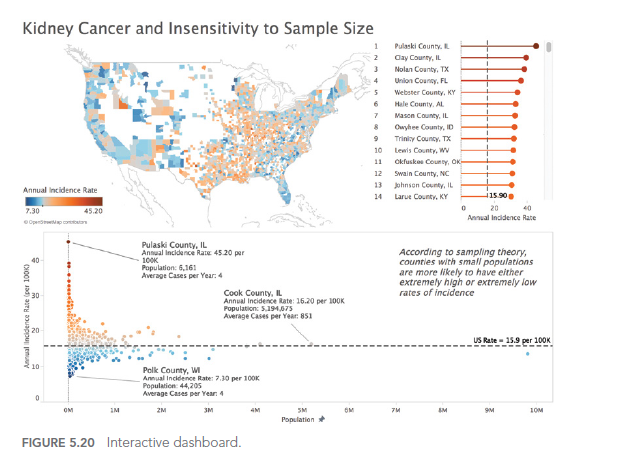
Also, note how in the scatterplot below the map, the marks form a funnel shape, with less populated counties (on the left) more likely to deviate from the reference line (the overall U.S. rate), and more populated counties like Chicago, L.A. and New York are more likely to be close to the overall reference line.
One final observation: if you hover over a county with a small population in the interactive online version, you’ll notice that the average number of cases per year is extremely low, sometimes 4 cases or less. A small deviation – even just 1 or 2 cases – in a subsequent year will pull a county from the bottom of the list to the top, or vice versa.
In the next article, we’ll explore the 5th type of error we may encounter when using data to illuminate the world around us: Analytical aberrations.
This article is heavily inspired by the book “Avoiding Data pitfalls – How to steer clear of common blunders when working with Data and presenting Analysis and visualization” written by Ben Jones, Founder and CEO of Data Litercy, WILEY edition. We recommend this excellent read!
You can find all the topics covered in this series here: https://www.businesslab.mu/blog/artificial-intelligence/data-7-pitfalls-to-avoid-the-introduction/