Data Warehouses vs Data Lakes: a comparative dive into the Tech World
In the ever-evolving world of technology, two terms have been making waves: Data Warehouses and Data Lakes. Both are powerful tools for data storage and analysis, but they serve different purposes and have unique strengths and weaknesses. Let’s dive into the world of data and explore these two tech giants.
Data Warehouses have been around for a while, providing a structured and organized way to store data. They are like a well-organized library, where each book (data) has its place. Recent advancements have made them even more efficient. The convergence of data lakes and data warehouses, for instance, has led to a more unified approach to data storage and analysis. This means less data movement and more efficiency – a win-win!
Moreover, the integration of machine learning models and AI capabilities has automated data analysis, providing more advanced insights. Imagine having a personal librarian who not only knows where every book is but can also predict what book you’ll need next!
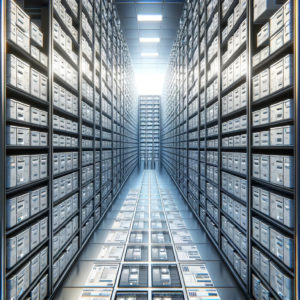
However, every rose has its thorns. Data warehouses can be complex and costly to set up and maintain. They may also struggle with unstructured data or real-time data processing. But they shine when there is a need for structured, historical data for reporting and analysis, or when data from different sources needs to be integrated and consistent.
On the other hand, Data Lakes are like a vast ocean of raw, unstructured data. They are flexible and scalable, thanks to the development of the Data Mesh. This allows for a more distributed approach to data storage and analysis. Plus, the increasing use of machine learning and AI can automate data analysis, providing more advanced insights.
However, without proper management, data lakes can become « data swamps », with data becoming disorganized and difficult to find and use. Data ingestion and integration can also be time-consuming and complex. But they are the go-to choice when there is a need for storing large volumes of raw, unstructured data, or when real-time or near-real-time data processing is required.
In depth
DATA WAREHOUSES
Advancements
1. Convergence of data lakes and data warehouses: This allows for a more unified approach to data storage and analysis, reducing the need for data movement and increasing efficiency.
2. Easier streaming of real-time data: This allows for more timely insights and decision-making.
3. Integration of machine learning models and AI capabilities: This can automate data analysis and provide more advanced insights.
4. Faster identification and resolution of data issues: This improves data quality and reliability.
Setbacks
1. Data warehouses can be complex and costly to set up and maintain.
2. They may not be suitable for unstructured data or real-time data processing.
Best scenarios for implementation
1. When there is a need for structured, historical data for reporting and analysis.
2. When data from different sources needs to be integrated and consistent.
DATA LAKES
Advancements
1. Development of the Data Mesh: This allows for a more distributed approach to data storage and analysis, increasing scalability and flexibility.
2. Increasing use of machine learning and AI: This can automate data analysis and provide more advanced insights.
3. Tools promoting a structured dev-test-release approach to data engineering: This can improve data quality and reliability.
Setbacks
1. Data lakes can become « data swamps » if not properly managed, with data becoming disorganized and difficult to find and use.
2. Data ingestion and integration can be time-consuming and complex.
Best scenarios for implementation
1. When there is a need for storing large volumes of raw, unstructured data.
2. When real-time or near-real-time data processing is required.
In conclusion, both data warehouses and data lakes have their own advantages and setbacks. The choice between them depends on the specific needs and circumstances of the organization. It’s like choosing between a library and an ocean – both have their charm, but the choice depends on what you’re looking for. So, whether you’re a tech enthusiast or a business leader, understanding these two tools can help you make informed decisions in the tech world. After all, in the world of data, knowledge is power!