Informed decision-making: fast and effective
« Promptness in decision-making is the pillar of success, but data insight is the foundation »
This adage perfectly sums up the subject of effective and rapid decision-making, which in the majority of businesses is based on data.
In today’s business world, data has become the fuel that drives strategic decision-making. From planning day-to-day operations to developing long-term strategies, businesses are now leveraging data to guide their choices and improve their overall effectiveness.
Here’s how data-driven decisions can radically transform your business. Whether you’re a leader in your sector or expanding into a new market, you’ll inevitably have to make strategic decisions that will affect your business.
Knowing that the wrong decision can have serious consequences for your project, and even for your company, it’s essential to have the right processes, decision-making tools and, above all, data.
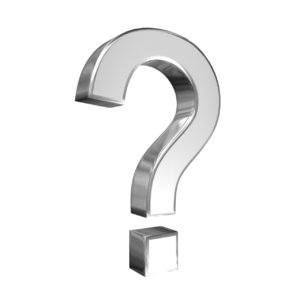
Accuracy and relevance
Data-driven decisions are based on tangible, factual information, eliminating guesswork and hunches that are often prone to error. By using accurate, up-to-date data, businesses can make more informed and relevant decisions, reducing the risk of costly errors.
Identifying trends
By analysing large data sets, businesses can identify significant trends and recurring patterns. This enables them to anticipate market changes, identify new opportunities and stay ahead of the competition.
Personalising customer experiences
Customer behaviour data enables businesses to create personalised, tailored experiences. By understanding individual customer needs and preferences, businesses can offer better-tailored products and services, boosting customer loyalty and satisfaction.
Using technology to accelerate & optimise the process
Operational data enables companies to optimise their internal processes. By identifying inefficiencies and bottlenecks, companies can make precise adjustments to improve productivity, reduce costs and increase overall operational efficiency.
Data processing technologies such as artificial intelligence (AI), machine learning and predictive analytics can accelerate the decision-making process by automating repetitive tasks and providing actionable insights in real time. Advanced algorithms can detect subtle patterns in data, helping decision-makers to make better and faster decisions.
Data-driven decisions: the key to agility & agile decision-making
With real-time access to data, businesses can make decisions faster and more agilely. Using real-time dashboards and analysis, decision-makers have the information they need to react quickly to market changes and new opportunities.
Informed decision-making depends on access to accurate, up-to-date data. Companies that invest in data collection, analysis and visualisation systems are better equipped to make rapid, informed decisions. By exploiting available data, they can quickly assess market trends, understand customer needs and identify opportunities for growth.
Speed without compromising quality
While speed is essential in a competitive business environment, this does not mean sacrificing the quality of decisions. Data provides an objective framework on which to base choices, reducing the risk of costly errors associated with impulsive or ill-informed decision-making. By combining speed and accuracy, businesses can make effective decisions while maintaining a high level of quality and relevance.
The importance of a data culture
Beyond tools and technologies, informed decision-making depends on an organisational culture that values data and fosters collaboration. Companies that foster a data culture are better equipped to collect, analyse and effectively use information to make decisions. By encouraging transparency, communication and collaboration, these companies can fully exploit the potential of data to drive innovation and growth.
Conclusion
By adopting a data-driven approach, businesses can transform the way they make decisions, moving from an approach based on intuition to one based on tangible, verifiable data. As a result, they can improve operational efficiency, drive growth and maintain competitiveness in the ever-changing marketplace. Ultimately, businesses that fully embrace data-driven decision-making are better positioned to thrive in the modern economy.
Informed, data-driven decision-making offers an undeniable competitive advantage in the modern business environment. By combining speed and efficiency with the accuracy of data, businesses can adapt quickly to market changes, seize opportunities and maintain their position as leaders in their sector. By investing in advanced data processing technologies and fostering a data-driven culture within the organisation, businesses can successfully navigate an ever-changing world and thrive in the face of uncertainty.